
RI Seminar
October
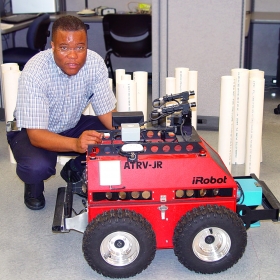
4:00 pm to 12:00 am
Event Location: Mauldin Auditorium (NSH 1305)
Bio: Emmanuel G. Collins, Jr. received the Ph.D. degree in Aeronautics and Astronautics from Purdue University in 1987. He worked for seven years in the Controls Technology Group at Harris Corporation, Melbourne, FL before joining the Department of Mechanical Engineering at the Florida A&M University – Florida State University College of Engineering, Tallahassee, FL, where he currently serves as John H. Seely Professor and Director of the Center for Intelligent Systems, Control and Robotics (CISCOR). His current research interests include navigation and control of autonomous vehicles (ground, water and air) in extreme environments and situations, multi-robot and human-robot cooperation, and control of aero-propulsion systems. He is the author of over 200 technical publications in control and robotics and served for several years as an associate editor of the IEEE Transactions on Control System Technology. He is the recipient of the Honorary Superior Accomplishment Award from NASA Langley Research Center for “contributions in demonstrating active control of flexible spacecraft.”
Abstract: Motion planning with dynamic models is an area of research that is still in its infancy. Progress in this field requires the synergy of dynamic modeling, control technology, and artificial intelligence (AI). This seminar discusses the potential uses of dynamic models in planning and reviews the ongoing journey of the speaker and his research group to develop paradigms and technology that enable these models to be used effectively in planning. The discussion on dynamic modeling focuses on skid-steered vehicles, which are very difficult to model due to the complex tire/terrain interaction. Particular attention is given to the use of feedback control to minimize the sensitivity of the models to uncertainties. Model predictive control (MPC) is seen as an existing control paradigm that appears to be effective for planning. However, inadequacies (i.e., slow computational speeds and convergence to local minima) in the optimization approaches traditionally associated with MPC are highlighted. Hence, we propose to combine MPC with sampling and A*-type optimization, which have proven effective for real-time optimization in AI and robotics. The result is an algorithm that we call Sampling Based Model Predictive Control (SBMPC) that has several existing planning algorithms as special cases. The seminar concludes with a discussion of the implications of SBMPC outside of robotic planning and an analysis of future research directions.