
RI Seminar
November
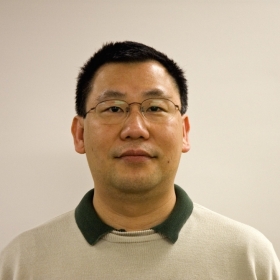
3:30 pm to 12:00 am
Event Location: NSH 1305
Bio: Sing Bing Kang received his Ph.D. in robotics from CMU in 1994. He is Principal Researcher at Microsoft Corporation and adjunct faculty member of the School of Interactive Computing at Georgia Tech. His interests are image-based modeling as well as image and video enhancement. Sing Bing has co-edited two books in computer vision (“Panoramic Vision” and “Emerging Topics in Computer Vision”), and co-authored a book on image-based rendering. He has served as area chair for the three major computer vision conferences (ICCV, CVPR, and ECCV), and was program co-chair for ACCV’07 and CVPR’09. He has also served as papers committee member for SIGGRAPH’07, SIGGRAPH Asia’08, and SIGGRAPH’09, as well as papers advisory board member for SIGGRAPH Asia’08 and ’09. He is currently an Associate Editor for IEEE TPAMI and Associate Editor-in-Chief for IPSJ Transactions On Computer Vision and Applications.
Abstract: Achieving realism is one of the major goals of computer graphics, and many approaches, ranging from physics-based modeling of light and geometry to image-based rendering, have been proposed. Realistic imagery has revolutionized the design process, allowing for the presentation and exploration of unbuilt scenes. Unfortunately, creating new content for realistic rendering (in the context of games, for example) remains tedious and time-consuming. In this talk, I will introduce the idea of “sketching reality”, the process of converting a freehand sketch into a realistic-looking model. To illustrate this idea, I will describe how we convert sketches of architectural designs and trees to their respective 3D models. The key to generating plausible-looking models is the use of prior knowledge of buildings and trees. Prior knowledge on buildings is in the form of type of architecture and its associated detailed geometry and texture, while that for trees is the association of shape to tree growth parameters. In each case, we use a probabilistic framework to infer the most likely model given the sketch. I will show a variety of results to validate our techniques.