
VASC Seminar
May
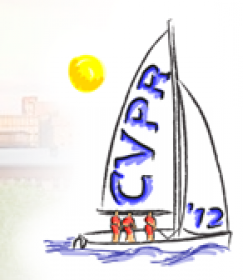
12:00 pm to 2:00 pm
Event Location: NSH 3305
Abstract: **Pizza Lunch will be served at 1:00pm**
Talk #1 (starting at 12:00pm)
The M-Best Mode Problem: Extracting Diverse M-Best Solutions from Graphical Models
Dhruv Batra, Research Assistant Professor
TTI-C
Abstract:
A large number of problems in computer vision, computational biology and robotics can formulated as the search for the most probable state under a discrete probabilistic model — known as the MAP inference problem in Markov Random Fields (MRFs). In many situations, one could benefit from additional solutions with high probability. Current methods for computing the M most probable configurations produce solutions that tend to be very similar to the MAP solution and each other. This is often an undesirable property.
In this talk, I will describe our recent work on the M-Best Mode problem, which involves finding a /diverse/ set of highly probable solutions under a discrete probabilistic model. Extracting the top M modes of the distribution allows us to better understand and exploit the beliefs that our model holds.
Joint work with Greg Shakhnarovich (TTIC), Abner Guzman-Rivera (UIUC) and Payman Yadollahpour (TTIC).
Host: Abhinav Gupta
Appointments: abhinavg@cs.cmu.edu
Talk #2 (starting at 1:15pm) – Internal CVPR Practice Talk
Model Recommendation for Action Recognition
Pyry Matikainen, Ph.D. Candidate
Robotics Institute, CMU
Abstract:
Simply choosing one model out of a large set of possibilities for a given vision task is a surprisingly difficult problem, especially if there is limited evaluation data with which to distinguish among models, such as when choosing the best “walk” action classifier from a large pool of classifiers tuned for different viewing angles, lighting conditions, and background clutter. In this paper we suggest that this problem of selecting a good model can be recast as a recommendation problem, where the goal is to recommend a good model for a particular task based on how well a limited probe set of models appears to perform. Through this conceptual remapping, we can bring to bear all the collaborative filtering techniques developed for consumer recommender systems (e.g., Netflix, Amazon.com). We test this hypothesis on action recognition, and find that even when every model has been directly rated on a training set, recommendation finds better selections for the corresponding test set than the best performers on the training set.
Talk #3 (starting at 1:45pm) – Internal CVPR Practice Talk
Occlusion Reasoning for Object Detection under Arbitrary Viewpoint
Ed Hsiao, Ph.D. Candidate
Robotics Institute, CMU
Abstract:
We present a unified occlusion model for object instance detection under arbitrary viewpoint. Whereas previous approaches primarily modeled local coherency of occlusions or attempted to learn the structure of occlusions from data, we propose to explicitly model occlusions by reasoning about 3D interactions of objects. Our approach accurately represents occlusions under arbitrary viewpoint without requiring additional training data, which can often be difficult to obtain. We validate our model by extending the state-of-the-art LINE2D method for object instance detection and demonstrate significant improvement in recognizing texture-less objects under severe occlusions.