
VASC Seminar
September
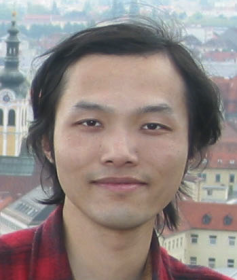
2:00 pm to 3:00 pm
Event Location: NSH 1305
Bio: Xiaofeng Ren is a research scientist at Intel Labs and an affiliate assistant professor at the University of Washington. His research interests are broadly in the areas of computer vision and its applications, including image features, grouping and segmentation, object recognition, and video analysis. His current focus is on understanding and solving computer vision problems in everyday life settings. He received his Ph.D. from University of California, Berkeley and his B.S. from Zhejiang University. Prior to joining Intel in 2008, he was on the research faculty of Toyota Technological Institute at Chicago.
Abstract: A visual processing pipeline typically consists of several stages, where the first stage computes representations of local image regions. While most research in vision studies later stages and relies on standard features such as SIFT, I will show that it pays off to be a friend with pixels: by formulating descriptors as measuring patch similarities, we derive a flexible framework called kernel descriptors that learns to capture local image characteristics. Kernel descriptors significantly outperform SIFT and the state of the art on a variety of classification benchmarks, and are particularly useful for working with RGB-D (Kinect) data for which we have less understanding. When combined with hierarchical segmentation, kernel descriptors improve scene labeling
accuracy by a large margin without need for sophisticated contextual models. In a parallel line of work on unsupervised feature learning, we show that local features can also be effectively learned through sparse coding, which leads to not only competitive results on recognition but also surprising advances on lower level problems such as contour detection.