
VASC Seminar
March
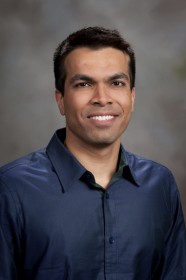
3:00 pm to 5:00 pm
Event Location: NSH 1109
Bio: Dhruv Batra is an Assistant Professor at the Bradley Department of Electrical and Computer Engineering at Virginia Tech, where he leads the VT Machine Learning & Perception group. He is a member of the Virginia Center for Autonomous Systems (VaCAS) and the VT Discovery Analytic Center (DAC). Prior to joining VT, he was a Research Assistant Professor at Toyota Technological Institute at Chicago (TTIC), a philanthropically endowed academic computer science institute located in the campus of University of Chicago. He received his M.S. and Ph.D. degrees from Carnegie Mellon University in 2007 and 2010 respectively, advised by Tsuhan Chen. In past, he has held visiting positions at Cornell University, at the Machine Learning Department at CMU, and at CSAIL MIT. His research interests lie at the intersection of machine learning, computer vision, and AI, with a focus on developing scalable algorithms for learning and inference in probabilistic models for holistic scene understanding. He has also worked on other topics such as interactive co-segmentation of large image collections, human body pose estimation, action recognition, depth estimation, and distributed optimization for inference and learning in probabilistic graphical models. He was a recipient of Carnegie Mellon Dean’s Fellowship (2007), Google Faculty Research Award (2013), Army Research Office (ARO) Young Investigator Program (YIP) award (2014), the National Science Foundation (NSF) CAREER award (2014), and Virginia Tech CoE Outstanding New Assistant Professor award (2015). His research is supported by NSF, ARO, ONR, Amazon, Google, Microsoft, and NVIDIA.
Abstract: What does a young child or a high-school student with no knowledge of probability do when faced with a problem whose answer they are uncertain of? They make guesses. Modern machine perception algorithms (for object detection, pose estimation, or semantic scene understanding), despite dealing with tremendous amounts of ambiguity, typically do not.
In this talk, I will describe a line of work in my lab where we have been developing machine perception algorithms that output not just a single-best solution, rather a /diverse/ set of plausible guesses. I will discuss inference in graphical models (because that’s how all good talks ought to start), connections to submodular maximization over a “doubly-exponential” space (because why not), and show results on problems such as semantic segmentation, pose estimation, and prepositional phrase attachment resolution in image captions (to convince you that this stuff is actually useful). Following my own advice, I will talk about other “multiple diverse” projects in my lab (including deep learning, of course).