
PhD Thesis Proposal
November
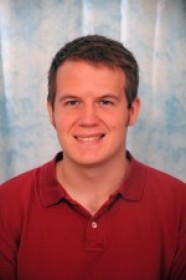
10:00 am to 12:00 am
Event Location: GHC 4405
Abstract: Given a single image of a scene, humans have few issues answering questions about the 3D structure like “is this facing upwards?” even though mathematically speaking this should be impossible. We have similarly have few issues accounting for this 3D structure in answering viewpoint independent questions like “is this the same carpet as the one in your office?”, even if the carpets were viewed from different views and have no pixels in common.
At the heart of the issue is that images are the result of two phenomena: the underlying 3D shape, which we call the 3D structure, and viewpoint-invariant textures that are applied on this shape, which we call the style. In the 3D world, these phenomena are distinct, but when we observe the world, they become mixed. Although the identity of both structure and style gets lost in the process, if we know about regularities in both phenomena, we can narrow down the possible combinations that could have produced our image.
This thesis aims to better enable computer to understand images in a 3D way by factoring the image into 3D structure and style. The key is that we can take advantage of regularity in both phenomena to inform our interpretation. For instance, we do not expect carpet texture on ceilings or 75 degree angles between walls. By using regularities, especially ones discovered from large-scale data, we can winnow away the possible combinations of 3D structure and style that could have produced our image and produce better and richer interpretations.
We first introduce a number of new ways to obtain this factorization in the form of mid-level bottom up cues, physical constraints, and the use of human-centric constraints. In proposed work, we aim to tackle: (1) unsupervised factoring of 3D structure and style by leveraging the regularity of human scenes; this lets us learn a model for single image 3D without ever seeing a single explicit 3D label; (2) the learning of constraints on style by large-scale factorization of Internet images; (3) the estimation of human affordances from a single image as source of complementary constraints on 3D structure.
Committee:Abhinav Gupta, Co-chair
Martial Hebert, Co-chair
Deva Ramanan
William T. Freeman, Massachusetts Institute of Technology
Andrew Zisserman, Oxford University