
PhD Thesis Proposal
February
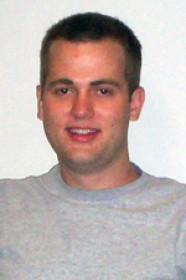
10:00 am to 12:00 am
Event Location: GHC 4405
Abstract: Each year, hybrid vehicles command a larger portion of total vehicles on the road. These vehicles combine multiple sources of energy, such as batteries and gasoline, which have different strengths and weaknesses. Active management of these energy sources can increase vehicle efficiency, longevity, or performance. Optimizing energy management is highly sensitive to upcoming power loads on the vehicle, but conventional control policies only react to the present state. These policies are computed at design-time and do not adapt to individual drivers. However, advancements in cheap sensing and computation have enabled on-board learning and optimization that was previously impossible.
In our prior work, we developed a real-time controller that learns and exploits the common patterns and routes of an individual driver. Energy management is optimized using data-driven predictions of upcoming power load. These predictions are computed using recorded data from prior observed trips. The vehicle iteratively adds new trips to its dataset, improving data coverage and performance over time. This proposal reports our simulated results using real-world driving data and poses our next extensions to verify and expand upon our findings.
We empirically evaluated our approach in a simulated battery-supercapacitor hybrid vehicle. The optimization task was to leverage the supercapacitor buffer to reduce current-squared served by the battery pack. We achieved performance within 10% of the optimal upper bound calculated using a priori knowledge of the upcoming loads. This suggests our data-driven predictive approach could be used for other optimization tasks in hybrid vehicle energy management. We propose to implement our system on multiple physical vehicles with different optimization goals. We will document challenges, evaluate performance, and provide a methodology for real-world adoption in vehicles. Additionally we propose to theoretically characterize the algorithm and quantify its longterm impact. Finally, we will develop a map representation of the data for visualization and analysis.
Committee:Illah Nourbakhsh, Chair
Jeremy Michalek
Jeff Schneider
Rahul Mangharam, University of Pennsylvania