
PhD Thesis Proposal
July
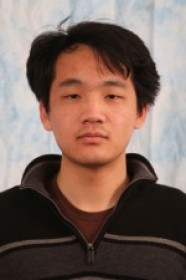
9:00 am to 12:00 am
Event Location: NSH 1305
Abstract: Perception and state estimation are critical robot competencies that remain difficult to harden and generalize. This is due in part to the incredible complexity of modern perception systems which are commonly comprised of dozens of components with hundreds of parameters overall. Selecting a configuration of parameters relies on a human’s understanding of the parameters’ interaction with the environment and the robot behavior, which we refer to as the “context.” Furthermore, evaluating the performance of the system entails multiple empirical trials, which often poorly predict the generality of the system.
We depart from the conventional wisdom that perception systems must generalize to be successful and instead suggest that a perception system need only do well in situations it encounters over the course of its deployment. This thesis proposes that greater overall perceptual generality can be achieved by designing perception systems that adapt to their local contexts by re-selecting perception system parameters. Towards this end, we have completed work on improving stochastic model fidelity and discuss our proposed work on applying reinforcement learning techniques to learn parameter selection policies from perceptual experience.
Committee:George Kantor
Sebastian Scherer
Katharina Muelling
Ingmar Posner, University of Oxford