
PhD Thesis Proposal
May
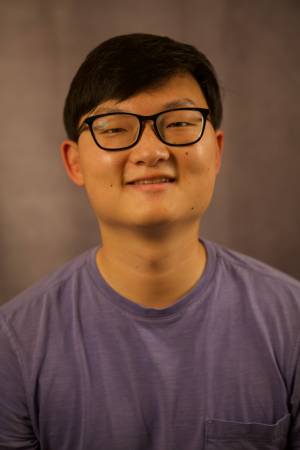
Abstract:
For intelligent agents (e.g. robots) to be seamlessly integrated into human society, humans must be able to understand their decision making. For example, the decision making of autonomous cars must be clear to the engineers certifying their safety, passengers riding them, and nearby drivers negotiating the road simultaneously. As an agent’s decision making can be captured by its reward function, we focus on teaching agent reward functions to humans.
Through reasoning that resembles inverse reinforcement learning (IRL), humans naturally infer reward functions that underlie demonstrations of decision-making. Thus agents can teach their reward functions through demonstrations that are informative for IRL. However, we critically note that IRL does not consider the difficulty for a human to learn from each demonstration. Thus, this thesis proposes to augment IRL with human modeling and teaching strategies to provide demonstrations at the right level of informativeness and difficulty for human understanding.
We first consider the problem of teaching reward functions through select demonstrations. We use scaffolding to convey demonstrations that gradually increase in informativeness and difficulty and ease the human into learning. And in calculating a demonstration’s informativeness, we leverage the fact that an informative demonstration is one that meaningfully differs from the human’s expectations (i.e. counterfactuals) of what the agent will do given their current understanding of the agent’s decision making.
We secondly consider the problem of testing, in which the agent asks humans to predict its behavior in new environments. We demonstrate two ways of measuring the difficulty of a test for a human. First, we posit that the difficulty of a test correlates directly to the answer’s informativeness at revealing the agent’s reward function. Second, we condition the difficulty of a test on the human’s current beliefs of the reward function, estimating the proportion of the human’s beliefs that would yield the correct behavior.
Finally, we propose new contributions to both the teaching and testing research thrusts. The work thus far has only taught low dimensional reward functions and we propose a method for teaching high dimensional reward functions to increase generalizability. And though tests have only been used for assessment thus far, we proposed to leverage the “testing effect” in the education literature to teach using well-selected tests as well.
Thesis Committee Members:
Reid Simmons, Co-chair
Henny Admoni, Co-chair
David Held
Scott Niekum, University of Texas at Austin