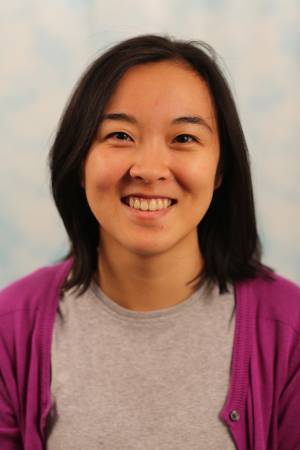
Abstract:
Two major obstacles for safe control and planning are (1) scaling to high-dimensional systems and (2) handling uncertain systems. This is problematic because such systems are ubiquitous in practice: e.g. drones with unknown drag, manipulators carrying unknown packages. In this proposal, we aim to address both challenges. At the control level, we have synthesized empirically safe controllers at higher dimensions (10D) than is possible with formal methods (6-8D). We have also synthesized robust-adaptive safe controllers, which can account for uncertainty without producing over-cautious behavior. In our proposed work, we consider safe planning for the target application of truck loading. Our goal is to design planners for real-time collision avoidance in cluttered environments. These planners should account for torque limits, singularities, and model uncertainty.
Thesis Committee Members:
John Dolan, Co-chair
Changliu Liu, Co-chair
Maxim Likhachev
Sylvia Herbert, UCSD