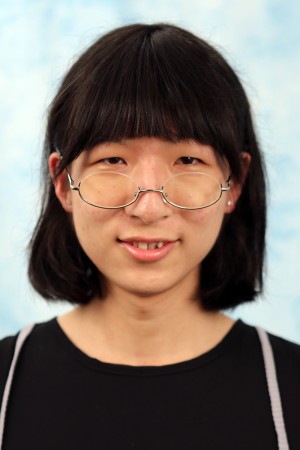
Carnegie Mellon University
Abstract:
3D multi-object tracking (MOT) is a key component of a perception system for autonomous driving. Due to recent progress in 3D object detection in the context of autonomous driving, recent work in 3D MOT primarily focuses on online tracking with the use of a tracking-by-detection pipeline. In this talk, we introduce a new 3D MOT evaluation metric that takes into account detection score uncertainty to provide a more holistic measure of performance. We also describe a new method for 3D MOT that learns discriminative relational features and takes into account features of neighboring objects to improve 3D MOT performance. Finally, we conclude with ideas from our on-going work on learning better representations for multi-object scene understanding in the context of the perception and prediction pipeline for autonomous systems.
Committee:
Kris Kitani (advisor)
Martial Hebert
David Held
Peiyun Hu