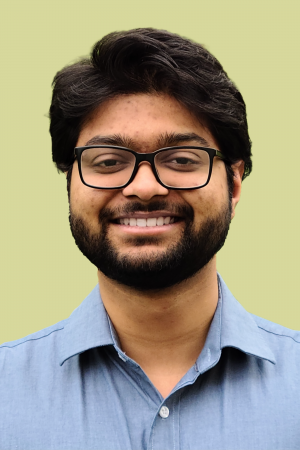
Abstract:
State estimation is a fundamental component of embodied perception. Contemporary autonomous vehicle (AV) benchmarks have advanced techniques for training 3D detectors, particularly on large-scale data. Notably, although prior work has nearly solved 3D object detection for a few common classes (e.g., pedestrian and car), detecting many rare classes in-the-tail (e.g., debris and stroller) remains challenging. We point out that fine-grained tail class accuracy is particularly improved via multi-modal fusion of RGB images with LiDAR; simply put, fine-grained classes are challenging to identify from sparse (LiDAR) geometry alone, suggesting that multi-modal cues are crucial to long-tailed 3D detection. We delve into a simple late-fusion framework that ensembles independently trained uni-modal LiDAR and RGB detectors. Importantly, such a late-fusion framework allows us to leverage large-scale uni-modal datasets (with more examples for rare classes) to train better uni-modal RGB detectors, unlike prevailing multimodal detectors that require paired multi-modal training data.
Furthermore, embodied agents must also forecast the behavior of others for safe navigation. Although precise state estimation requires both object detection (to understand the current position of all objects) and forecasting (to understand the future position of all objects), these two problems are largely studied in isolation by the community. We reframe forecasting as the task of future object detection, allowing us to repurpose mature detection machinery for end-to-end perception. Instead of predicting the current frame object locations and forecasting forward in time, we directly predict future object locations and backcast to determine where each trajectory began.
Lastly, we propose future work that unifies 3D perception across both indoor and outdoor environments. Notably, current approaches for indoor and outdoor perception use different representations (e.g. volumetric vs. bird’s-eye-view), evaluation metrics (3DAP vs. NDS), and evaluation protocols (e.g. scene-level vs. frame-level evaluation). Concretely, we propose methods, metrics, and datasets to jointly address indoor and outdoor perception from images and videos in-the-wild.
Thesis Committee Members:
Deva Ramanan, Chair
Shubham Tulsiani
Katerina Fragiadaki
Sanja Fidler, University of Toronto
Georgia Gkioxari, California Institute of Technology