
VASC Seminar
November
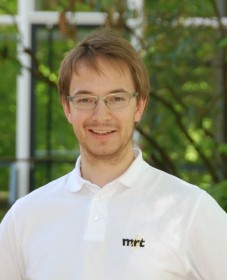
3:00 pm to 4:00 pm
Event Location: NSH 1507
Bio: Andreas Geiger studied computer science and mathematics at Karlsruhe Institute of Technology (KIT) in Germany. During his studies he spent 6 months at EPFL (working with Pascal Fua and Vincent Lepetit) and 6 months at MIT (working with Raquel Urtasun and Trevor Darrell). Currently he is a 4th year doctoral candidate at KIT under the supervision of Christoph Stiller (KIT) and Raquel Urtasun (TTI-C). His research interests are in computer vision and machine learning, with a focus on vision-based 3D scene understanding.
Abstract: Navigating a car savely through complex environments is considered a relatively easy task for humans. Computer algorithms, however, can’t nearly match human performance and often rely on 3D laser scanners or detailed maps. The reason for this is that the level and accuracy of current computer vision and scene understanding algorithms is still far from that of a human being. In this talk I will argue that pushing these limits requires solving a set of core computer vision problems, ranging from low-level tasks (stereo, optical flow) to high-level problems (object detection, 3D scene understanding). First, I will introduce the KITTI datasets and benchmarks with accurate ground truth for evaluating stereo, optical flow, SLAM and 3D object detection/tracking on realistic video sequences. Results from state-of-the-art algorithms reveal that methods ranking high on established datasets such as Middlebury perform below average when being moved outside the laboratory to the real world. Second, I will propose a novel generative model for 3D scene understanding that is able to reason jointly about the scene layout (topology and geometry of streets) as well as the location and orientation of objects. By using context from this model, performance of state-of-the-art object detectors in terms of estimating object orientation can be significantly increased. Finally, I will give an outlook on how prior information in form of large-scale community-driven maps (OpenStreetMap) can be used in the context of 3D scene understanding.