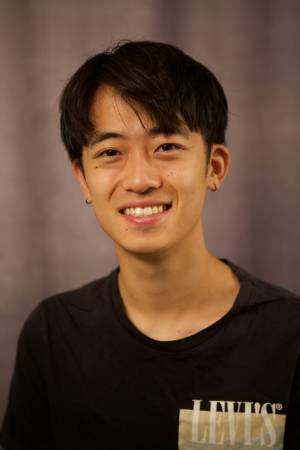
Abstract:
Task-oriented grasping requires robots to reason not only about object geometry, but also about the function and semantics of object parts in context. While large language models (LLMs) offer powerful commonsense knowledge, they lack grounding in physical geometry. This talk explores how symbolic object representations can bridge that gap, enabling LLMs to guide grasp selection in a zero-shot setting. We present two complementary approaches: the first, ShapeGrasp, constructs a geometric graph of object parts, which is semantically augmented through a multi-stage reasoning process. The second, SemanticCSG, takes a semantic-first approach, generating abstract hypotheses that are optimized to align with the observed 3D geometry of novel object instances. Through extensive real-world experiments across varied objects, tasks, and viewpoints, we show that grounding language-based reasoning in structured geometry yields robust, interpretable, and generalizable task-oriented grasping, outperforming prior baselines.
Committee:
Prof. Katia Sycara (advisor)
Prof. Shubham Tulsiani
Brian Yang