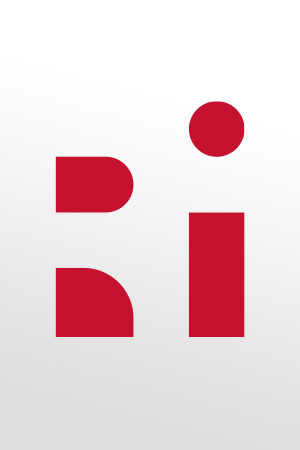
Abstract:
3D scene understanding from 2D video is essential for enabling advanced applications such as autonomous driving, robotics, virtual reality, and augmented reality. These fields rely on accurate 3D spatial awareness and dynamic interaction modeling to navigate complex environments, manipulate objects, and provide immersive experiences. Unlike 2D, 3D training data is much less abundant, which makes feedforward data-driven trackers less accurate. On the other hand, current optimization-based 3D and 4D reconstruction methods, such as NeRF-based approaches and Gaussian Splatting-based methods, are limited to static or simple object dynamics and struggle with multi-object scenes, fast motions, sparse views, and occlusions typical in real-world settings as they do not utilize data-driven priors. In this thesis, we hope to combine data-driven and optimization-based methods to understand 3D geometry and motion in videos.
First, we introduce Dreamscene4D, a framework that combines data-driven object-tracking priors, generative image priors, and 4D Gaussian Splatting for reconstructing monocular multi-object videos with complex and fast motion. DreamScene4D decomposes video scenes into individual objects and backgrounds, reconstructing their complete 3D geometry and motion. It combines the speed of data-driven priors with the precision of optimization-based techniques, outperforming state-of-the-art methods like dynamic NeRF and Gaussian Splatting in complex, real-world videos.
Next, we discuss how to use optimization-based techniques to produce 3D tracking data to develop 3D world models, in terms of data-driven 3D point forecasters that condition on actions and predict distributions over future 3D point motions. To enhance the diversity of the training data, we use a modified version of 4D Gaussian Splatting to distill 3D data from multi-view real-world videos. By jointly training on simulated and real-world data, our model can accurately predict future object configurations based on action inputs.
Finally, we outline future directions for advancing 4D video understanding and forecasting, aiming to improve both accuracy and applicability to in-the-wild videos.
Thesis Committee Members:
Katerina Fragkiadaki, Chair
Kris Kitani
Shubham Tulsiani
Kosta Derpanis, York University