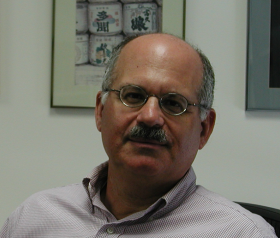
3:00 pm to 4:00 pm
Event Location: NSH 1507
Bio: Larry S. Davis received his B.A. from Colgate University in 1970 and his M. S. and Ph. D. in Computer Science from the University of Maryland in 1974 and 1976 respectively. From 1977-1981 he was an Assistant Professor in the Department of Computer Science at the University of Texas, Austin. He returned to the University of Maryland as an Associate Professor in 1981. From 1985-1994 he was the Director of the University of Maryland Institute for Advanced Computer Studies. He was Chair of the Department of Computer Science from 1999-2012. He is currently a Professor in the Institute and the Computer Science Department, as well as Director of the Center for Automation Research. He was named a Fellow of the IEEE in 1997 and of the ACM in 2013.
Prof. Davis is known for his research in computer vision and high performance computing. He has published over 100 papers in journals and 200 conference papers and has supervised over 35 Ph. D. students. During the past ten years his research has focused on visual surveillance and general video analysis. He has served as program or general chair for most of the field’s major conferences, including the 5’th International Conference on Computer Vision, the 2004 Computer Vision and Pattern Recognition Conference, the 11’th International Conference on Computer Vision held in 2006, and the 2010 Computer Vision and Pattern Recognition Conference. He is the co-General chair of the 2013 International Conference on Computer Vision to be held in Sydney in December 2013.
Abstract: This talk will introduce, in a collection of vignettes, a variety of current research projects at Maryland on visual recognition. The unifying theme is the use of attributes to boost recognition/retrieval performance in a variety of ways. As an appetizer, I will discuss coupling classification and ranking for attribute consistent person re-identification. The main course discusses three applications of predictable discriminative binary codes – to choose unlabeled samples from a large pool to augment small labeled training sets and improve recognition performance; for multi-modal hashing of images and text; and for performing domain adaptation. If time and space permits, desert will include a discussion of “vision as a service.” The research represents work done by several of my graduate students – Sameh Khamis, Mohammad Rastegari, Jonghyun Choi, Fatemeh Mir Rashid and Brandyn White.