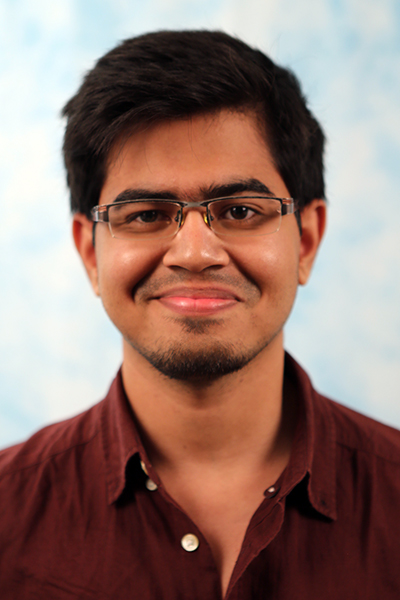
3:00 pm to 4:30 pm
NSH 4305
Title: Human Torso Pose Forecasting for the Real World
Abstract:
Anticipatory human intent modeling is important for robots operating alongside humans in dynamic or crowded environments. Humans often telegraph intent through posture cues, such as torso or head cues. In this paper, we describe a computationally lightweight approach to human torso pose recovery and forecasting with a view towards limited sensing for easy on-board deployment. Our end-to-end system combines RGB images and point cloud information to recover 3D human pose, bridging the gap between learning-based 2D pose estimation methods and the 3D nature of the environment that robots and autonomous vehicles must reason about, with minimum overhead. In addition to pose recovery, we use a simple filter-and-fit method to forecast torso pose. We focus on rapidly generating short horizon forecasts, which is the most relevant scenario for autonomous agents that iteratively alternate between data gathering and planning steps in highly dynamic environments. While datasets suited to benchmarking multi-person 3D pose prediction in real-world scenarios are scarce, we describe an easily replicable evaluation method for benchmarking in a near real-world setting. We then assess the pose estimation performance using this evaluation procedure. Lastly, we evaluate the forecasting performance quantitatively on the Human3.6M motion capture dataset. Our simple 3D pose recovery method adds minimum overhead to 2D pose estimators, with comparable performance to 3D pose estimation baselines from a computer vision alternative. Further, our uncomplicated forecasting algorithm outperforms more complex recurrent neural network methods while also being faster on the torso pose forecasting task.
Committee:
Aaron Steinfeld (Co-chair)
Henny Admoni (Co-chair)
Kris Kitani
Ishani Chatterjee