
VASC Seminar
October
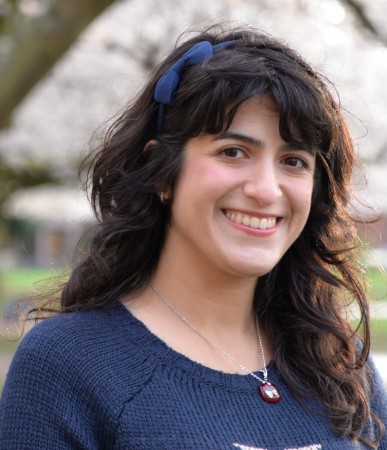
3:00 pm to 4:00 pm
GHC 6501
Abstract: In recent years, there have been great advances in policy learning for goal-oriented agents. However, there are still major challenges brought by real-world constraints for teaching highly generalizable and versatile robot policies in a cost efficient and safe manner. In this talk, I will argue that instead of aiming to teach large motion repertoires to robots for doing diverse tasks, we need to couple autonomous policy learning with understanding of the real-world semantics. Such a learning approach will help robots gain highly generalizable policies that can transfer and adapt to unstructured novel environments. I will discuss how we can harness the simulation capabilities and computer vision techniques for sim-to-real transfer of robotic policies to drastically diverse settings. I will then demonstrate results for manipulation and navigation using cheap mobile robots such as drones as well as industrial robotic arms.
Bio: Fereshteh Sadeghi is a PhD candidate in Computer Science at University of Washington, advised by Sergey Levine and Larry Zitnick. Fereshteh is a recipient of the NVIDIA Graduate Fellowship and her research is focused on developing learning algorithms that combine perception and control in autonomous embodied systems. She is interested in how learning can be used to enable machines acquired complex behavioral skills that can generalize to unstructured real world settings. In her PhD, she has developed techniques for learning highly generalizable robot controllers in simulation for efficient transfer and adaptability to the real world. She has spent time as a research intern at Allen Institute for AI and has recently been a student researcher at Google Brain Robotics.