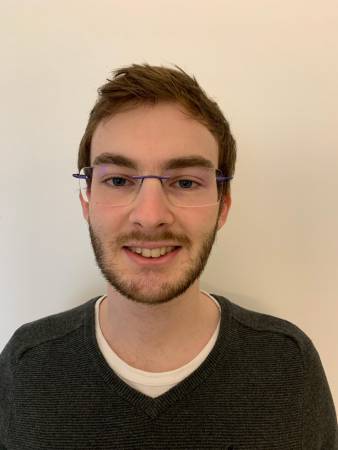
Abstract:
Optimization and machine learning-based methods are increasingly critical in enhancing the autonomy, efficiency, and overall return on investment (ROI) of small, resource-constrained spacecraft. By enabling more effective decision-making, adaptive control, and robust state estimation, these techniques expand mission capabilities while operating within strict mass, power, and computational limitations. This thesis builds on previous contributions to the development of small spacecraft systems, with a particular focus on applying optimal control, state estimation, and machine learning to improve spacecraft autonomy across guidance, navigation, and control (GNC) as well as perception and decision-making processes. Achieving higher levels of autonomy in small spacecraft presents novel challenges across multiple subsystems, including thermal regulation, control, and navigation. Increased onboard computation for autonomy leads to significantly higher heat dissipation, requiring innovative thermal management strategies to ensure reliable operation, particularly in planetary and deep-space environments where temperatures are extreme. In attitude and orbit control, propellant-free methods such as magnetorquer-based Lyapunov control and differential drag formation flying provide viable solutions for precise maneuvering within resource-constrained platforms. Similarly, in spacecraft navigation, visual-inertial techniques offer a means of improving state estimation in GPS-denied environments by integrating computer vision with inertial measurements. Machine learning methods further enhance these capabilities by improving feature extraction and uncertainty-aware landmark detection, enabling more robust localization in scenarios with limited prior information. Building on these foundations, proposed research will focus on developing onboard georectification methods for satellite imagery, enabling direct mapping of captured images to known planetary reference frames. Furthermore, optimization and machine learning techniques will be refined to improve landmark detection and state estimation, further enhancing spacecraft autonomy in dynamic and uncertain environments. These advancements will contribute to increasing the scientific and operational value of small satellite missions by enabling more precise navigation, mapping, and autonomous decision-making in space exploration.
Thesis Committee Members:
Zac Manchester (Chair)
Red Whittaker
Brandon Lucia
Andrew Horchler (Astrobotic)