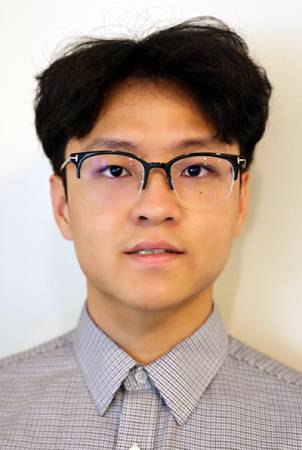
Abstract:
Recent advancements in vision-language foundation models, exemplified by GPT4-Vision and DALL-E 3, have significantly transformed both research and practical applications, ranging from professional assistance to content creation. However, aligning them precisely with specific user goals presents a notable challenge. This thesis introduces innovative strategies for improving this alignment. I will first introduce our novel cross-modal adaptation framework, utilizing textual/audio data to tailor foundational models such as CLIP more effectively to tasks such as visual recognition. Next, I will present an optimization approach based on ChatGPT for automatically aligning popular proprietary (black-box) models, like DALL-E 3, to better meet user needs. Lastly, I will share our latest efforts to assess and enhance model fidelity in target tasks requiring advanced visio-linguistic reasoning over compositions of objects, attributes, and their relations.
Committee:
Prof. Deva Ramanan (advisor)
Prof. Deepak Pathak
Prof. Graham Neubig (LTI)
Mihir Prabhudesai (RI PhD student)