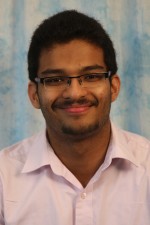
Carnegie Mellon University
10:00 am to 11:00 am
GHC 8102
Abstract:
Optical Coherence Tomography (OCT) and Ultrasound (US) are non-ionizing and non-invasive imaging modalities that are clinically used to visualize anatomical structures in the body. OCT has been widely adopted in clinical ophthalmology due to its micron-scale resolution to visualize in-vivo structures of the eye. Ultra-High Frequency Ultrasound (UHFUS) captures images of tissue at a depth of ~1cm with 30 micron resolution.
One key application area for OCT is assessing the stem cell distribution residing inside the Palisades of Vogt (POV) in the limbus. The limbus is located at the intersection of the clear cornea and the white sclera in the eye. Another application area is identifying corneal tissue interfaces for surgical procedures, such as Laser In-Situ Keratomileusis (LASIK). As for UHFUS, the key application in this thesis is vascular measurements, including monitoring for possible chronic rejection of hand transplants.
Achieving higher resolutions with OCT and UHFUS increases the speckle noise during imaging due to smaller resolution cells. In addition to the speckle noise present at every pixel, other localized imaging artifacts, such as shadowing and specular saturation artifacts, substantially diminish the visibility of tissue interface boundaries. These boundaries and edges are crucial for diagnosing particular pathological conditions or diseases, and for developing a pre-operative surgical plan. Moreover, abrupt tissue motion obfuscates the analysis of image sequences, including volumetric sweeps. An ideal approach to circumventing these issues would be validated across multiple devices and acquisition parameters, produce measurements that are clinically/surgically relevant, and be amenable to real-time implementation.
The main contribution of this thesis is in developing classical computer vision-based and deep learning-based approaches to address the problems faced in these clinical application areas. We have also developed a hybrid framework that removes OCT speckle noise from the air gap using a learning-based method, thereby dramatically improving the performance of classical approaches in situations where they previously failed. The presented classical and learned approaches all utilize consumer Graphics Processor Units (GPUs) for high-speed parallel computation to advance the clinical capabilities of OCT and UHFUS.
In this thesis, we have enabled visualizing changes in tissue structures over time and have begun quantifying some of these changes as well. Our improved OCT volumetric reconstructions allow us to extract cross-sectional images of the Palisades of Vogt (POV) from OCT sequences acquired by different OCT scanners with different scan settings to quantify the POV structural changes over time. We have designed state-of-the-art deep learning-based methods for scanner-agnostic segmentation of multiple corneal tissue interfaces. We have developed the first artificial intelligence system for measuring the intimal vessel wall thickness in human subjects imaged using UHFUS. Our methods have identified salient regions of image sequences for additional analysis of application-specific features and quantitative metrics.
Thesis Committee Members:
John Galeotti, Chair
George Stetten
Ruslan Salakhutdinov
Ajay Gopinath, Abbott