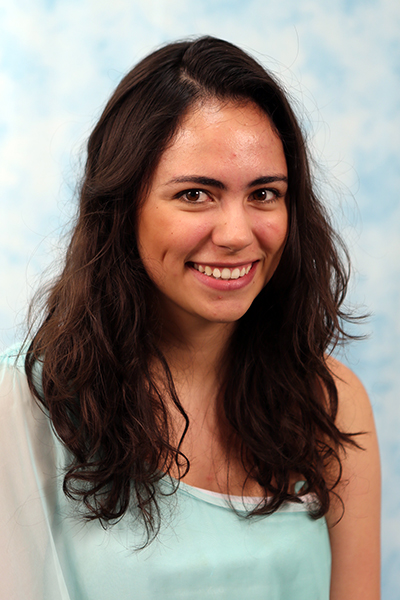
Abstract:
Medical emergencies demand rapid and accurate interventions to save lives. Severe injuries often require surgical care within the first 60 minutes when timely action significantly improves survival rates. However, limited resources, remote locations, and unpredictable conditions often obstruct access to advanced medical care during this critical period.
This thesis focuses on developing a medical force multiplier to improve emergency response capabilities, enabling effective care for critically injured individuals in diverse scenarios ranging from car accidents to large-scale conflicts. Leveraging Artificial Intelligence (AI), the work seeks to expand the capacity of emergency medical responders by facilitating efficient triage and clinical interventions in the field. Additionally, it explores the unique challenges AI encounters in real-world, unstructured environments, particularly in life-critical situations.
I propose that solving translational research problems involving AI in field care requires addressing three primary challenges: limited access to high-quality real-world data, constraints on computing capacity in portable systems, and the practical implications of using AI for life-critical decision making. Specifically, AI systems must avoid exacerbating risk to patients by recognizing when uncertainty in data and models may render interventions unsafe.
To address the challenge of limited data, we propose algorithms that augment existing datasets while incorporating domain knowledge. Our focus is on models optimized for deployment in portable systems that can function effectively in diverse and resource-constrained environments. Additionally, to ensure safe and reliable decision-making in life-critical scenarios, we implement uncertainty assessment techniques.
While sensing vital signs in the field, noise and environmental factors often obscure the signals. We propose to leverage Bayesian aggregation to capture and eliminate systematic signatures of measurement disturbances, and to fuse evidence from multiple sensors, to boost accuracy of vital sign assessments.
Thesis Committee Members:
Artur Dubrawski, Chair
Jean Oh
Fernando de la Torre
Daniel McDuff, Google
Laura Brattain, University of Central Florida