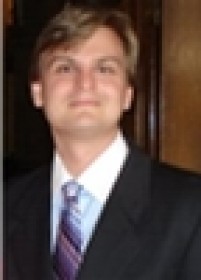
11:00 am to 12:00 am
Event Location: NSH3305
Bio: Geoffrey A. Hollinger is a Postdoctoral Research Associate in the Robotic Embedded Systems Laboratory and Viterbi School of Engineering at the University of Southern California. He is currently interested in adaptive sensing and distributed coordination for robots operating with limited communication. He has also worked on multi-robot search at Carnegie Mellon University, personal robotics at Intel Research Pittsburgh, active estimation at the University of Pennsylvania’s GRASP Laboratory, and miniature inspection robots for the Space Shuttle at NASA’s Marshall Space Flight Center. He received his Ph.D. (2010) and M.S. (2007) in Robotics from Carnegie Mellon University and his B.S. in General Engineering along with his B.A. in Philosophy from Swarthmore College (2005).
Abstract: There is growing interest in the use of robotic vehicles for inspection tasks, such as evaluation of aging facilities, surveying of disaster sites (e.g., the Fukushima nuclear plant), and sweeping for maliciously placed mines and explosive devices. Autonomous robots are uniquely suited for such tasks because they are capable of entering areas that may be dangerous or inaccessible to human operators. The increasing capabilities of the robots themselves enable more sophisticated decision making techniques that optimize information gathered and adapt as new information is received. The question becomes: how do we develop path planning and decision making techniques capable of dealing with the noisy sensing, mobility restrictions, and environment uncertainty characteristic of such tasks?
This talk considers the problem of robotic inspection with specific application to observing underwater structures and environments (e.g., ship hulls, oil platforms, and sea floors) with an Autonomous Underwater Vehicle (AUV). In this application domain, the goal is to develop an accurate 3D model and to detect any anomalies that may represent deformations or foreign objects. I will discuss techniques for constructing 3D meshes from sonar-derived point clouds, and I will introduce a measure of reconstruction uncertainty through non-parametric Bayesian regression. Such uncertainty modeling provides novel cost functions for planning the path of the robot that allow for formal analysis through connections to submodular optimization and active learning. Leveraging these formal connections, I will show that the benefit of adaptive planning over non-adaptive planning can be bounded through the characterization of provable adaptivity gaps. Such analysis gives us tools for determining when to act adaptively and also provides insight into the underlying structure of active sensing problems. Finally, I will present experiments on AUVs performing ship hull inspection and lake bed mapping that demonstrate the high performance of the proposed solutions versus the state of the art in robot path planning.