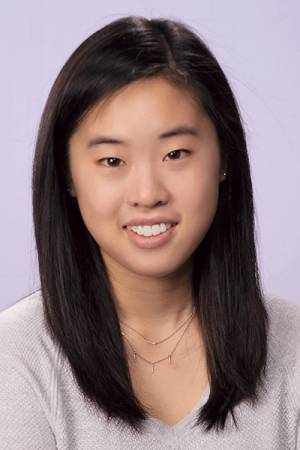
Abstract:
Ensuring pedestrian safety in dynamic environments is a key challenge for autonomous systems, particularly in dynamic, multi-agent environments. Trajectory forecasting plays a central role in enabling these systems to anticipate pedestrian behaviors and respond appropriately. This thesis addresses three core limitations in trajectory forecasting systems which impede safe and robust trajectory forecasting: inadequate evaluation protocols that fail to capture realistic multi-agent interactions, the lack of safety-critical vehicle-pedestrian interaction data, and limited progress in leveraging the gamut of multimodal data sources to make forecasting decisions.To address the first issue, we found that methods are able to achieve state-of-the-art prediction error despite producing forecasts with low social understanding,. We introduced joint error metrics over the traditionally-used marginal error metrics for model training and evaluation, leading to decreased collision predictions and better group coherence predictions. We also highlighted limitations in a public egocentric-perspective trajectory forecasting benchmark, where forecasting evaluation was entangled with odometry estimation evaluation. Disentangling the two problems provided clearer evaluations of model performance. To address the second issue, we built and leveraged a virtual reality system to collect safety-critical pedestrian-vehicle interaction data safely and efficiently, filling long-tail gaps in existing datasets. Finally, to address the third limitation, we explored using human pose keypoints extracted from visual data to inform trajectory forecasting, finding that higher-quality keypoints led to better forecasting performance. We also propose two avenues of further work: leveraging environmental data such as obstacles in trajectory forecasting, and ego-centric perspective vehicle intention forecasting from only RGB images using foundation models and 3d scene understanding methods.Together, this research aims to advance pedestrian safety by integrating reliable evaluation protocols, comprehensive datasets, and better methods into trajectory forecasting.
Thesis Committee Members:
Kris Kitani, Co-chair
Deva Ramanan, Co-chair
Aaron Steinfeld
Hamid Rezatofighi, Monash University