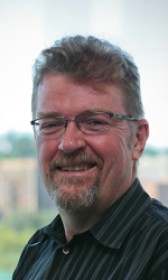
3:00 pm to 4:00 pm
Event Location: NSH 1507
Bio: Dr. Robert F. Murphy is the Ray and Stephanie Lane Professor of Computational Biology and Head of the Computational Biology Department in the School of Computer Science at Carnegie Mellon University. He is also Professor of Biological Sciences, Biomedical Engineering, and Machine Learning at Carnegie Mellon, Honorary Professor of Biology at the Albert Ludwig University of Freiburg, Germany, and a Fellow of the American Institute for Medical and Biological Engineering. Dr. Murphy has served as President of the International Society for Advancement of Cytometry and as a member of the NIH National Advisory General Medical Sciences Council,and the NIH Council of Councils. Dr. Murphy’s career has centered on combining fluorescence-based cell measurement methods with quantitative and computational methods. His group at Carnegie Mellon did extensive work on the application of flow cytometry to analyze endocytic membrane traffic beginning in the early 1980’s. In the mid 1990’s, his group pioneered the application of machine learning methods to high-resolution fluorescence microscope images depicting subcellular location patterns, and was the first to demonstrate superior machine performance in interpreting diverse patterns in biological images. His current research interests include machine learning of image-derived models of cell organization, automated detection of protein location changes during oncogenesis, and active machine learning approaches to experimental biology.
Abstract: Machine learning methods are critically needed for building systems models of cell and tissue behavior and for studying their perturbations. Such models require accurate, cell-type specific information about the shape and distributions of subcellular structures and the distributions of proteins, RNAs and other macromolecules in order to be able to capture and simulate cellular spatiotemporal dynamics. We have developed tools to build generative models of cell organization directly from microscope images of many cells. Generative models are capable of producing new instances of a pattern that are expected to be drawn from the same underlying distribution as those it was trained with. Our open source system, CellOrganizer (http://CellOrganizer.org), currently contains components that can build probabilistic generative models of cell, nuclear and organelle shape, organelle position, and microtubule distribution. These models capture heterogeneity within cell populations, and can be dependent upon each other and can be combined to create new higher level models. Recent work has focused on learning relationships between different cellular structures and on constructing models of the dynamics of changes in cell organization.