
MSR Thesis Defense
June
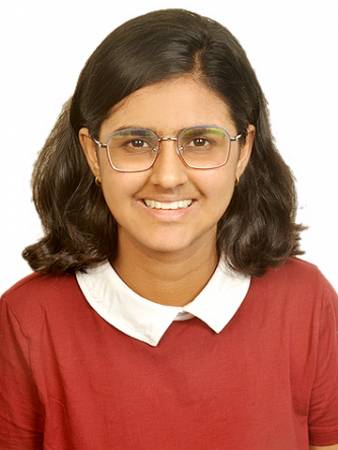
Abstract:
This research introduces CBGT-Net, a neural network model inspired by the cortico-basal ganglia-thalamic (CBGT) circuits in mammalian brains, which are crucial for critical thinking and decision-making. Unlike traditional neural network models that generate an output for each input or after a fixed sequence of inputs, CBGT-Net learns to produce an output once sufficient evidence for action is accumulated from a stream of observed data. For each observation, CBGT-Net generates a vector representing the amount of evidence for each potential decision, accumulates this evidence over time, and makes a decision when the accumulated evidence surpasses a predefined or dynamically learned threshold.
We evaluate the proposed model on various image classification tasks, where the model must predict image categories based on a stream of partially informative visual inputs. Our results demonstrate that CBGT-Net offers improved accuracy and robustness compared to models trained to classify from a single image, as well as models utilizing an LSTM layer or a ViT-style transformer to classify from a fixed sequence of image inputs. Additionally, we introduce a novel dataset for classification based on sequential image data of urban city buildings. This dataset provides multi-view images of 3D building assets on fire, categorized into five stages of fire severity.
Committee:
Prof. Katia Sycara (advisor)
Prof. Steven Chase
Ini Oguntola