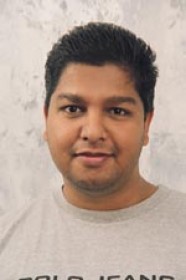
12:00 pm to 12:00 am
Event Location: NSH 1507
Abstract: When interacting with our surroundings, our actions are highly structured. This structure is a consequence of the purposefulness of human behavior — we tend to do similar things in similar circumstances. Artificial systems must develop an understanding of the underlying dynamics that encode this structure to understand human actions in a scene. In this thesis, we propose analytical models coupled with closed-form estimation that encode the structured dynamics of human motion and investigate their use for action understanding and 3D reconstruction of the human posture from monocular videos.
Human motion can be categorized at different levels of granularity. At the finest level is instantaneous motion, which is defined as motion between two consecutive image frames. The next level of granularity is short-term motion, which is defined as motion across a few image frames. At the next higher level level of granularity is micro-action, which is defined as the smallest unit of motion with semantic meaning to humans. Human motion exhibits rich interaction between these different granularities and developing statistical models that capture the dynamics of individual granularities and their mutual interactions is key to developing a computational understanding of human motion.
At the finest level of motion granularity is instantaneous motion which is defined as motion between two consecutive image frames. Analytical models for instantaneous motion estimation should not only accurately estimate imaged human motion but should also capture the articulated nature of human motion. Humans are articulated objects with constraints on angles of revolute limb joints and therefore motion between two consecutive frames is correlated. We develop an articulated motion estimation algorithm to capture this fundamental nature of human motion. In addition, we explicitly take into account the uncertainty associated with articulation localization in the image plane to compute motion estimates.
The next level of motion granularity is short-term motion which is defined as motion across a few image frames. Due to the purposeful nature of the human action, which induces similar motion in similar circumstances across people; short-term human motion, therefore usually admits a collection of locally linear subspaces that serve as representative models of motion. A collection of locally linear subspaces allows us to linearly regularize motion estimation algorithms over the nonlinear human motion manifold, thus avoiding the limitation of global models in previous literature that require commitment to a uniform dimensionality for the latent motion manifold.
The next higher level of motion granularity are micro-actions which are used to denote motion with semantic meaning to humans. In contrast to previous approaches that advocate the imposition of latent nonlinear priors to regularize the recovery of motion estimates, we propose to leverage motion capture based prior in a RANSAC-driven optimization scheme. In addition to recovering the motion estimates, we show how this approach can be used to perform not only action recognition but also to gain a 3D understanding of the micro-action by recovering camera position and orientation from monocular video; we investigate the relationship between these two tasks and how the recovery of one aids the other. It is our belief that by developing analytical models that capture the dynamics and the interactions between the different granularities of human motion, computers can perform analysis of human motion in monocular videos.
Committee:Takeo Kanade, Co-chair
Yaser Sheikh, Co-chair
Martial Hebert
Simon Baker, Microsoft Research
Tsuhan Chen, Cornell University