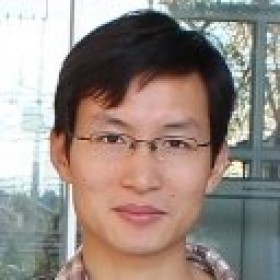
2:00 pm to 3:00 pm
Event Location: NSH 1507
Bio: Yang Wu received a BS degree and a Ph.D degree from Xi’an Jiaotong University in 2004 and 2010, respectively. From Sep. 2007 to Dec. 2008, he was a visiting student in the GRASP lab at University of Pennsylvania. From 2011 to 2014, he was a program specific researcher at the Academic Center for Computing and Media Studies, Kyoto University. Within this period, he was an invited academic visitor at the Big Data Institute of University College London from Jul. 2014 to Aug. 2014. He is currently an assistant professor of the NAIST International Collaborative Laboratory for Robotics Vision, Center for Frontier Science and Technology, Nara Institute of Science and Technology. His research is in the fields of computer vision, pattern recognition, and image/video search and retrieval, with particular interests in detecting, tracking and recognizing humans and generic objects. He is also interested in pursuing general data analysis models applicable to large data sets.
Abstract: Sparse representation (SR) models have been studied for a long time, showing great power in acquiring, representing, compressing, restoring and even classifying high-dimensional signals. Their success is largely due to the fact that many important classes of signals including audio and images have naturally sparse representations with respect to fixed bases (i.e., Fourier, wavelet, or concatenations of them) or just some representative samples in the domain-specific subspaces. Among the enthusiasm in believing the power of sparsity in classification tasks, there is a distinctive argument which is worth noticing: “it is the collaborative representation of the test sample using all the training samples that truly results in SRC’s success but not the sparsity of representation coefficients.” This argument has motivated our recent research on collaborative representation (CR) for classification. In this area, we have got some encouraging and inspiring results, especially on the problem of person re-identification. I will introduce our progress in developing collaborative representation models for this specific application and also show that how such models perform on other classification problems.