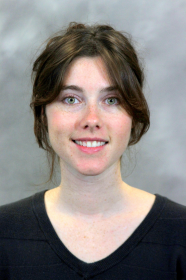
3:00 pm to 4:00 pm
Event Location: NSH 1507
Bio: Genevieve is a PhD Candidate in Computer Vision at Brown University. Her work on crowd-driven visual classification was recently awarded runner-up for Best Paper at the AAAI Conference on Human Computation (HCOMP). She built and maintains the SUN Attribute dataset, a widely used resource for scene understanding. Genevieve received her master’s in Electrical Machines from the University of Tokyo, where she won the ICEMS 2009 Outstanding Paper award for her work on transverse-flux motor design. She earned bachelor’s degrees in Electrical Engineering and Mathematics from the University of Arizona.
Abstract: Crowd annotated datasets have become a mainstay in computer vision, enabling some of the most significant discoveries of recent years. However, the research community has yet to fully exploit the full range of human intelligence available in the crowd. This talk demonstrates that, by going beyond naive annotation, researchers can access the vast potential of crowdsourcing. Using polling, active learning, intelligent annotation protocols, and other techniques we are able to leverage the crowd to discover a taxonomy of visual attributes, build detectors with minimal supervision, and economically label massive datasets.