
PhD Speaking Qualifier
April
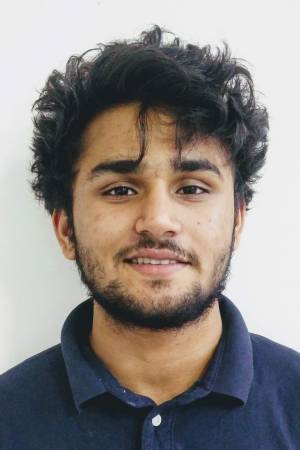
4:00 pm to 5:00 pm
NSH 3305
Abstract:
Computer Vision is Correspondence, correspondence, correspondence! Inspite of the singular definition of computer vision, we still have two broad categories of approaches in the literature. Generative Models, like Stable Diffusion, learn a correspondence between image and text modality, while learning a mapping from text to image. Discriminative Models, like CLIP, on the other hand learn the same correspondence , while learning a mapping from image to text. Now while both these models are learning the same correspondence, they end up modeling very different statistics of the same data distribution due to the opposite directionality of mapping. In this talk, I will explain how the features these methods learn are different and how they can be combined to improve each other’s performance. In this talk I’ll discuss three of my works, Diffusion-TTA, Diffusion-Classifier, and AlignProp. I’ll also discuss my ongoing work that builds on top of these ideas.
Committee:
Deepak Pathak
Katerina Fragkiadaki
Deva Ramanan
Russell Mendonca