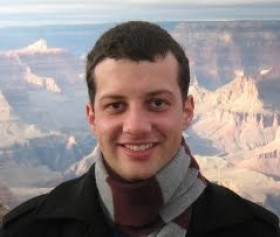
2:30 pm to 3:00 pm
Event Location: NSH 1507
Bio: Philipp is a postdoctoral researcher at UC Berkeley. He received his PhD from Stanford University in 2014 under the supervision of Vladlen Koltun. His main research interests lie in Computer vision, Machine learning and Computer Graphics. He is particularly interested in image segmentation and deep learning.
Abstract: In this talk, I’ll present an approach to learn a dense pixel-wise labeling from image-level tags. We phrase the problem as a constraint optimization in a Convolutional Neural Network (CNN). We use a novel loss function, to train a CNN using any set of linear constraints on the output space (i.e. predicted label distribution). Our loss formulation is easy to optimize and can be incorporated directly into standard stochastic gradient descent optimization. Extensive experiments demonstrate the generality of our new learning framework. The constrained loss yields state-of-the-art results on weakly supervised semantic image segmentation. We further demonstrate that adding slightly more supervision can greatly improve the performance of the learning algorithm.