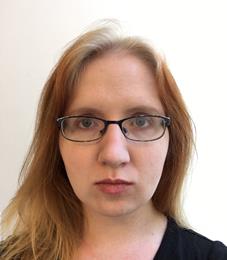
Abstract:
The ability for an agent to continuously learn new skills without catastrophically forgetting existing knowledge is of critical importance for the development of generally intelligent agents. Most methods devised to address this problem depend heavily on well-defined task boundaries which simplify the problem considerably. Our task-agnostic method, Self-Activating Neural Ensembles (SANE), uses a hierarchical modular architecture designed to avoid catastrophic forgetting without making any such assumptions. At each timestep, a path through the SANE tree is activated to determine the agent’s next action. During training, new nodes are created as needed, and only activated nodes are updated, ensuring that unused nodes remain unchanged. Thus, the system enables agents to leverage and retain old skills while growing and learning new ones. We demonstrate our approach on MNIST and a set of grid world environments, showing that SANE does not undergo catastrophic forgetting where existing methods do.
Committee:
Abhinav Gupta (advisor)
Chris Atkeson
Katerina Fragkiadaki
Anirudh Vemula