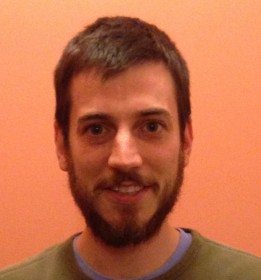
2:00 pm to 12:00 am
Event Location: NSH 3305
Abstract: In this thesis proposal, we describe a data-driven approach to leverage repositories of 3D models for scene understanding. Our ability to relate what we see in an image to a large collection of 3D models allows us to transfer information from these models, creating a rich understanding of the scene. We develop a framework for auto-calibrating a camera, rendering 3D models from the viewpoint an image was taken, and computing a similarity measure between each 3D model and an input image. We demonstrate this data-driven approach in the context of geometry estimation and show the ability to find the identities and poses of object in a scene. Additionally, we present a new dataset with annotated scene geometry. This data allows us to measure the performance of our algorithm in 3D, rather than in the image plane.
We also present a novel application of our geometry estimates, which utilize motion capture data to analyze the affordances of a scene. In addition, we propose a series of extensions built upon our existing geometry estimation algorithm to speedup computation, increase robustness and boost the overall performance of our system.
Committee:Martial Hebert, Chair
Alexei Efros
Abhinav Gupta
Derek Hoiem, University of Illinois at Urbana-Champa