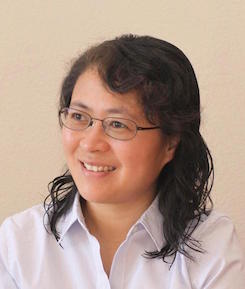
1:30 pm to 2:30 pm
GHC 6501
Abstract: Computer vision has advanced rapidly with deep learning, achieving above human performance on some classification benchmarks. At the core of the state-of-the-art approaches for image classification, object detection, and semantic/instance segmentation is sliding window classification, engineered for computational efficiency. Such piecemeal analysis of visual perception often has trouble getting details right and fails miserably with occlusion. Human vision, on the other hand, thrives on occlusion and excels at seeing both large and small, whole and parts at the same time. I will describe our work on affinity CNN, neural multigrid, unsupervised instance discrimination, and adaptive affinity fields, which pursues perceptual organization in a data-driven deep learning fashion, and in turn delivers more accurate and generalizing performance at image classification and segmentation.
Bio: Stella Yu received her Ph.D. from Carnegie Mellon University, where she studied robotics at the Robotics Institute and vision science at the Center for the Neural Basis of Cognition. She continued her computer vision research as a postdoctoral fellow at UC Berkeley, and then studied art and vision as a Clare Booth Luce Professor at Boston College, during which she received an NSF CAREER award. Dr. Yu is currently the Director of Vision Group at the International Computer Science Institute (ICSI) and a Senior Fellow at the Berkeley Institute for Data Science (BIDS) at UC Berkeley. Dr. Yu is interested not only in understanding visual perception from multiple perspectives, but also in using computer vision and machine learning to capture and exceed human expertise in practical applications. She has several postdoc openings for motivated computer vision researchers with strong math+coding skills.
Homepage: http://www.icsi.berkeley.edu/~stellayu