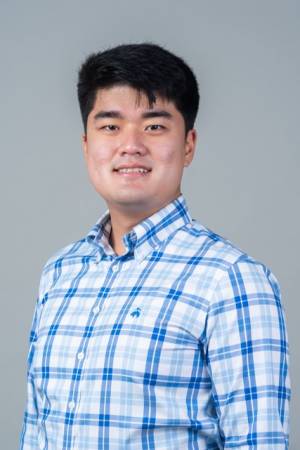
Deformation-Aware Manipulation: Compliant and Geometric Approaches for Non-Anthropomorphic Hands
Abstract:
Soft robot hands offer compelling advantages for manipulation tasks, including inherent safety through material compliance, robust adaptation to uncertain object geometries, and the ability to conform to complex shapes passively. However, these same properties create significant challenges for conventional sensing and control approaches. This talk presents approaches to bridging advances in geometric learning and non-anthropomorphic robot hands, enabling dexterous manipulation with soft and deformable manipulators. I will discuss three research directions: (1) exploiting mechanical intelligence by leveraging a soft robot’s inherent deformation for robust dynamic manipulation and safe physical human-robot interaction; (2) developing proprioceptive state representation and sensing methods including different modalities to capture complex deformation behaviors of soft hands; and (3) applying these tools toward learning to execute dexterous skills. I will discuss how these approaches collectively help enable deformation-aware dexterous manipulation, showing that by exploiting rather than avoiding compliance, we can create manipulation systems that are safe, robust, and dexterous. Finally, I will discuss ongoing and proposed work that extends these capabilities by developing methods to transfer manipulation skills from human demonstrations to non-anthropomorphic soft hands and explore transferring skills among robot hand designs toward exploring the question of how we might teach robots with different morphologies to perform dexterous tasks.
Thesis Committee:
Jean Oh (co-chair)
Jeffrey Ichnowski (co-chair)
Christopher G. Atkeson
Dorsa Sadigh (Stanford University)