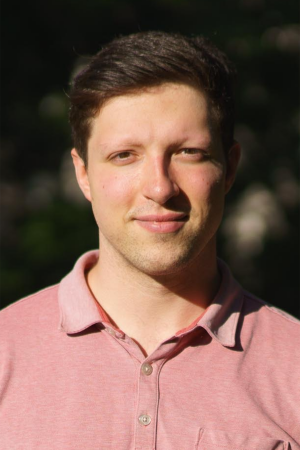
Abstract:
When solving a manipulation task like “put away the groceries” in real environments, robots must understand what *can* happen in these environments, as well as what *should* happen in order to accomplish the task. This knowledge can enable downstream robot policies to directly reason about which actions they should execute, and rule out behaviors that are unhelpful or impossible. However, inferring these properties directly from raw sensor observations presents a challenge: how can we get learning systems to map high-dimensional visual inputs to these precise geometric properties in a way that downstream policies can consume, while generalizing to novel environments with different object shapes, sizes, and classes?
In this talk, I will discuss two novel dense 3D visual representations we have proposed to accomplish high-precision inference of these task-relevant geometric properties, while generalizing to novel variations of objects and scenes. First, I will introduce 3D Articulation Flow (from the FlowBot3D system), a dense 3D flow field which can represent how an arbitrary number of articulated parts on an object are constrained to move (i.e. doors, drawers, etc.). Second, I will introduce how predicting dense, cross-object correspondences can enable precise goal inference in relative object placement tasks, which we call Task-Specific Cross-Pose (TAX-Pose). Finally, I’ll discuss some future directions for this line of work and how it might fit into long-horizon reasoning and manipulation tasks.
Committee:
David Held
Oliver Kroemer
Shubham Tulsiani
Yufei Ye