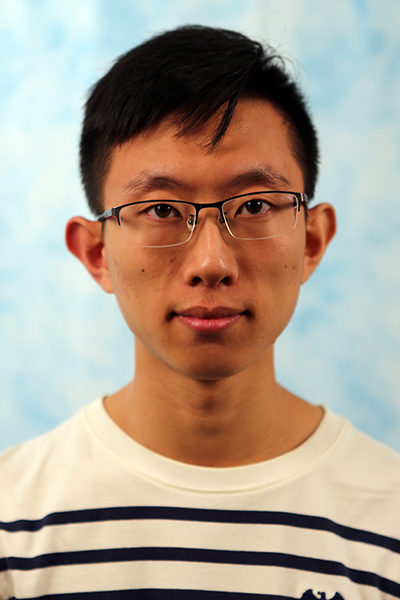
Abstract:
We study the problem of 3D reconstruction of {\em generic} and {\em deformable} objects and scenes from {\em casually-taken} RGB videos, to create a system for capturing the dynamic 3D world. Being able to reconstruct dynamic structures from casual videos allows one to create avatars and motion references for arbitrary objects without specialized devices, which is beneficial to VR and robotics applications. However, one fundamental challenge is the under-constrained nature of the problem: from limited 2D visual observations, there exist multiple interpretations of the geometries and motion of the 3D world. To constrain the problem, previous methods either take advantage of specialized sensors (e.g., synchronized multi-camera systems), or 3D shape templates (e.g., parametric human body models). However, neither of them scales robustly to diverse sets of objects in the wild, such as cats and dogs.
To design methods for dynamic 3D reconstruction without relying on specialized sensors or 3D shape templates, we first look at a simple form of the problem — given a single image or a pair of stereo images, how to estimate distributions over depth. Then we move to a more complex problem — given two consecutive frames of a video, how to estimate the geometry, dense 3D motion fields, and a rigid decomposition of the scene. We cast those low-level pixel prediction problems as supervised learning tasks, and design neural architectures that leverage volumetric representation and two-view geometry priors to improve robustness on out-of-distribution test data. Next, we look at the problem of nonrigid 3D shape estimation from one or multiple casually-captured videos. Our approach combines inverse-graphics optimization with generic data-driven priors (e.g., optical flow, feature correspondence, segmentation), and builds articulated 3D models from monocular RGB videos without using a pre-built shape template.
The proposed works aim to build a high-quality library of articulated shape and environment models from large-scale video collections, that can be replayed in a physics simulator. One challenge is how to decompose the foreground and background with high-precision for in-the-wild video footage. Another challenge comes from the inherent ambiguity of the monocular reconstruction task — how to constrain the reconstructions such that they are physically plausible. Lastly, we explore feed-forward inference methods for virtual avatar creation from images and videos.
Thesis Committee Members:
Deva Ramanan, Chair
Shubham Tulsiani
Jessica Hodgins
Yaser Sheikh
Angjoo Kanazawa, UC Berkeley