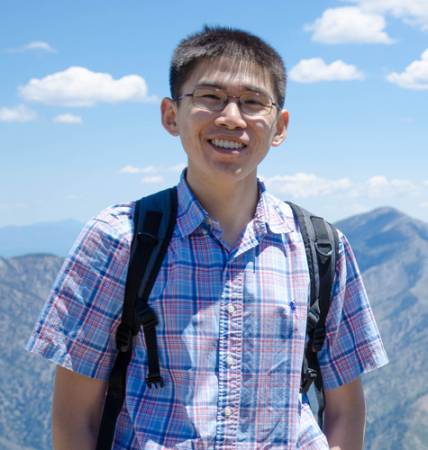
Abstract: Vision-language models pretrained on web-scale data have revolutionized deep learning in the last few years. They have demonstrated strong transfer learning performance on a wide range of tasks, even under the “zero-shot” setup, where text “prompts” serve as a natural interface for humans to specify a task, as opposed to collecting labeled data. These models are trained on “composite” data, such as visual scenes of multiple objects, or a sentence that describes that spatiotemporal event. However, it is not clear whether they do this by learning to reason over lower-level, spatio-temporal “primitive” concepts that humans naturally use to characterize these concepts, such as colors, shapes, or verbs that describe short actions. If they do so, it has important implications for the capacity of models to support compositional generalization, and for humans to interpret the reasoning procedures models undertake.
In this talk, I will present our recent attempts to answer this question. We study several representative vision-language (VL) models trained on images (e.g. CLIP) and videos (e.g. VideoBERT), and design corresponding “probing” frameworks to understand if VL pretraining: (1) improves lexical grounding, (2) encodes verb meaning, and (3) learns visually grounded primitive concepts. I will also discuss a direction to improve vision-language from unlabeled long videos, by learning temporal abstractions.
Bio: Chen Sun is an assistant professor of computer science at Brown University, studying computer vision, machine learning, and artificial intelligence. He is also a staff research scientist at Google, and has served as area chair for AAAI, CVPR, ECCV, etc. Chen received his Ph.D. from the University of Southern California in 2016, and bachelor degree from Tsinghua University in 2011. His research appeared in the CVPR 2019 best paper finalist.
Homepage: https://chensun.me/
Sponsored in part by: Facebook Reality Labs Pittsburgh