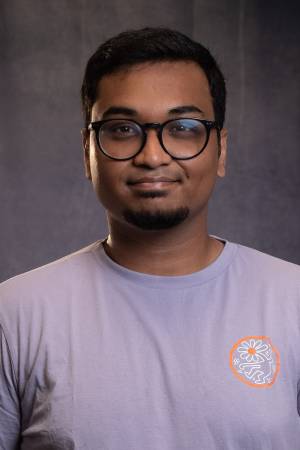
We tackle object importance estimation in a data-driven fashion and introduce HOIST – Human-annotated Object Importance in Simulated Traffic. HOIST contains driving scenarios with human-annotated importance labels for vehicles and pedestrians. We additionally propose a novel approach that relies on counterfactual reasoning to estimate an object’s importance. We generate counterfactual scenarios by modifying the motion of objects and ascribe importance based on how the modifications affect the ego vehicle’s driving. Strong performance on importance estimation, and an explainable nature make it ideal for driving assistance.
Next, we tackle driver’s situational awareness (SA) estimation. Situational Awareness is an internal human cognitive state, hence acquiring ground-truth awareness labels is challenging. We first propose a novel interactive labeling protocol that effectively captures dense, continuous SA labels and use it to collect an object-level SA dataset in a VR driving simulator. We formulate object-level driver SA prediction problem as a semantic segmentation problem. This formulation allows all objects in a scene at a timestep to be processed simultaneously, leveraging global scene context and local gaze-object relationships together. Our experiments show that this formulation leads to improved performance over common sense baselines and prior art on the SA prediction task.
Committee:
Prof. Andrea Bajcsy
Ravi Pandya