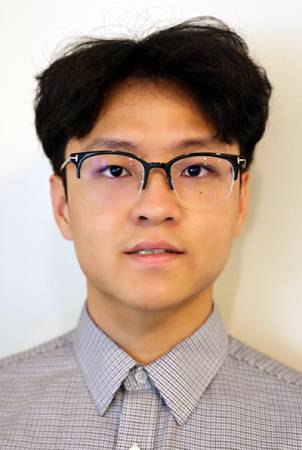
Abstract:
In this talk, we present our work on advancing Vision-Language Models (VLMs) beyond scaling laws through improved evaluation and (post-)training strategies. Our contributions include VQAScore, a state-of-the-art alignment metric for text-to-visual generation. We show how VQAScore improves visual generation under real-world user prompts in GenAI-Bench. Additionally, we explore training methods that leverage the language modality to enhance visual reasoning across discriminative, generative, open-source, and even proprietary VLMs. Lastly, we also show how benchmarking biases and limitations in VLMs provides insights that drive their improvements. The talk concludes with plans to extend this work to video-language models for enhanced spatial-temporal reasoning.
Thesis Committee Members:
Deva Ramanan, Chair
Deepak Pathak
Graham Neubig
Ali Farhadi, University of Washington, Allen Institute for AI