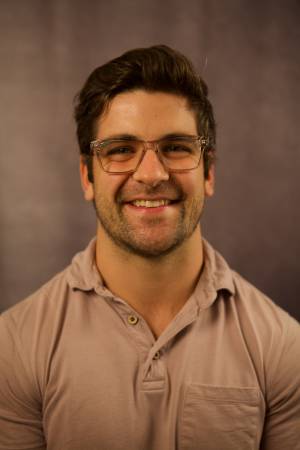
Abstract:
Teaching sessions between humans and robots will need to be maximally informative for optimal robot learning and to ease the human’s teaching burden. However, the bulk of prior work considers one or two modalities through which a human can convey information to a robot—namely, kinesthetic demonstrations and preference queries. Moreover, people will teach robots to perform a task according to their own, individual preferences, and as such, robots need to represent the task in a way that can handle this heterogeneity. This thesis addresses both needs. First, we investigated how an agent can maximize its information gain by actively selecting queries from a diverse set of interaction types (demonstrations, corrections, preference queries, and binary critiques). Second, we explored three reward function structures that could be used to model a human teacher’s preferences for how an agent should perform a task. Our evaluations showed that 1.) actively selecting from among a diverse set of interaction types yields faster, more robust learning, and 2.) an agent typically learns best when its reward function structure matches that of its teacher’s.
Committee:
Henny Admoni (co-advisor)
Oliver Kroemer (co-advisor)
Reid Simmons
Gokul Swamy