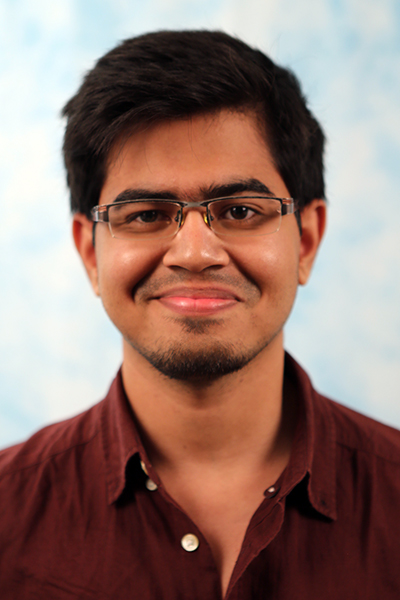
Eye Gaze for Intelligent Driving
Abstract:
Intelligent vehicles have been proposed as one path to increasing traffic safety and reducing on-road crashes. Driving “intelligence” today takes many forms, ranging from simple blind spot occupancy or forward collision warnings to distance-aware cruise and all the way to full driving autonomy in certain situations. Primarily, these methods are outward-facing and operate on information about the state of the vehicle and surrounding traffic elements. However, another less explored domain of intelligence is cabin-facing modeling information about the driver’s cognitive states.
In this thesis, we investigate the utility of a signal that can help us achieve cabin-facing intelligence: driver eye gaze. Eye gaze allows us to infer driver internal cognitive states and we explore how this can improve both autonomous driving methods and intelligent driving assistance. To enable this research, we first contribute DReyeVR, an open-source virtual reality driving simulator, which was designed with behavioural and interaction research priorities in mind but exists in the same experimental environments used by vehicular autonomy researchers, effectively bridging the two fields. We show how DReyeVR can be used to conduct psychophysical experiments by designing one to characterize the extent and dynamics of driver peripheral vision. We make good on the promise of bridging behavioural and autonomy research by using similar naturalistic driver gaze data to provide additional supervision to autonomous driving agents trained via imitation learning to mitigate causal confusion. We then turn to the assistive domain. First, we study false positives in a real-world dataset of forward collision warnings deployed in vehicles during a longitudinal study in-the-wild. We find that deploying FCWs purely based on scene physics without accounting for driver attention leads to overwhelming them with redundant alerts. We demonstrate a warning strategy that accounts for driver attention to explicitly model their hypothesis of other vehicles’ behaviour. Finally, we propose the shared awareness paradigm, a framework for continuously supporting driver situational awareness (SA) with an intelligent perception system. We track dynamic objects (e.g. vehicles, pedestrians etc.) and reason about them on two simultaneous fronts —drivers’ situational awareness and importance to driving safety for each object. To build the driver situational awareness model, we first collect data using a novel SA labeling method, to obtain continuous, per-object driver awareness labels along with their gaze, driving actions and the simulated world state. We use this data to learn a model that predicts drivers’ situational awareness of traffic elements given a history of their gaze and scene context. In parallel, we reason about the importance of objects in a counterfactual fashion by studying the impact of perturbing it on the ego vehicle’s motion plan. Finally, we put it all together, in an offline demonstration on replayed simulated drives to show how we could alert drivers of important objects they are unaware of.
We conclude by reflecting on how eye gaze can be used to model the internal cognitive states of human drivers, in service of improving both vehicle autonomy and driving assistance.
Thesis Committee Members:
Henny Admoni, Chair
David Held
Nik Martelaro
Chien-Ming Huang, Johns Hopkins