
Faculty Candidate
January
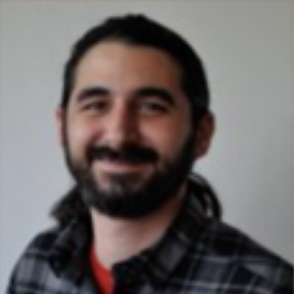
10:00 am to 11:30 am
Gates 6115
Areas of Interest:
Abstract
Robotic manipulation is a key functional requirement that is largely missing from the current state of the art regarding robots in unstructured environments. While models of manipulation phenomenon give us invaluable insights on various principles, many parameters (e.g. surface geometry, friction coefficients, contact locations) are difficult to accurately measure and implement into a model-based planning solution. From a control engineering perspective, manipulation planning algorithms are often open-loop, and need to make assumptions on missing or inaccurate model parameters. This talk will emphasize the importance of closing the loop in task space for accurate and robust robotic manipulation. Such an approach requires an interplay between vision-based control, system compliance and machine learning. Compliance in such a manipulation system helps to maintain stable contact, which is crucial for robust object handling, but introduces further modeling challenges and impairs accuracy. Closing the loop in task space with adaptive vision-based control techniques restores the lost accuracy and provides efficient execution of the task. Here, the role of machine learning is to guide the system towards the goal with high-level supervision, and prevent it from moving towards undesired states. These points will be covered for robotic grasping and dexterous manipulation with underactuated hands. In addition, the advantage of compliance during policy training will be discussed.
Bio
Berk’s research primarily focuses on vision-based robotic manipulation. He develops manipulation algorithms by combining techniques in computer vision, control theory, and machine learning. He completed his PhD at Delft University of Technology in The Netherlands, where he worked on active sensing algorithms aimed at increasing success rates of robotic grasping algorithms. He is one of the founders and the primary manager of Yale-CMU-Berkeley (YCB) benchmarking project, which provides a platform for the robotic manipulation community to develop shared benchmarking protocols. His other areas of interest are soft manipulation, active object recognition, tactile sensing and visual servoing. He is currently a post-doc in Yale University Grab Lab, where he works on vision-based dexterous manipulation with underactuated robot hands.
Host: Chris Atkeson
Admin Contact: Chris Downey cdowney@andrew.cmu.edu