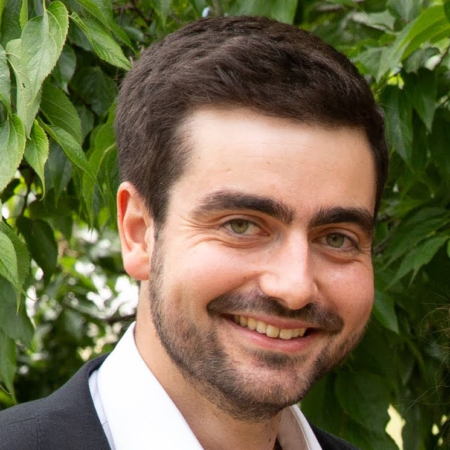
Karl Pertsch
UC Berkeley and Stanford
Monday, April 7
10:00 am to 11:00 am
Newell-Simon Hall 4305
10:00 am to 11:00 am
Newell-Simon Hall 4305
Faculty Candidate Talk: Karl Pertsch
Talk Title:
Unlocking Scalable Robot Learning in the Real World
Abstract:
Many domains of machine learning, from language modeling to computer vision, have recently undergone a shift towards generalist models, whose broad generalization abilities are fueled by large and diverse real-world training datasets and high-capacity model architectures. In robotics, however, it has been challenging to apply the same recipe: after all, we cannot easily scrape millions of hours of robot data from the internet and existing model architectures for scalable learning in vision or language modeling are not designed for the continuous control tasks we need to solve in robotics. In this talk, I will describe my work on unlocking scalable robot learning in the real world, focusing on the three key elements of any modern ML pipeline: data, models, and evaluations. I will discuss important differences between robotics and other machine learning domains, and describe how we can adapt scalable learning approaches for robotics. My work has enabled the construction of the largest robot learning datasets to date, and the training of generalist robot policies that can perform a range of manipulation tasks out of the box in unseen environments, simply by prompting them in natural language. I will close with a description of current limitations and open challenges towards building truly general robot control policies.
Bio:
Karl Pertsch is a postdoc at UC Berkeley and Stanford, jointly advised by Sergey Levine and Chelsea Finn. He also is a member of the technical staff at Physical Intelligence. His work focuses on building generalist robot policies that can solve a wide range of physical manipulation tasks in the real world. Karl obtained his PhD from USC, advised by Joseph Lim. During his PhD he interned at MetaAI and Google Brain. His work has been awarded the Best Conference Paper Award at ICRA’24 and two Outstanding Paper Award Finalists at CoRL’24.