
VASC Seminar
November
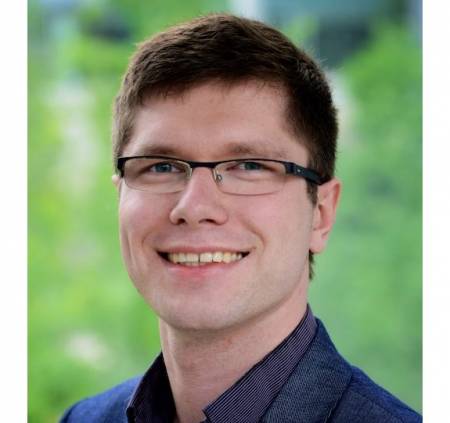
Abstract:
In this seminar I will demonstrate how synthetic data alone can be used to perform face-related computer vision in the wild. The community has long enjoyed the benefits of synthesizing training data with graphics, but the domain gap between real and synthetic data has remained a problem, especially for human faces. Researchers have tried to bridge this gap with data mixing, domain adaptation, and domain-adversarial training, but I will show that it is possible to synthesize data with minimal domain gap, so that models trained on synthetic data generalize to real in-the-wild datasets. I will describe how to combine a procedurally-generated parametric 3D face model with a comprehensive library of hand-crafted assets to render training images with unprecedented realism and diversity. I will show that models trained using synthetic data can both match real data in accuracy as well as open up new approaches where manual labelling would be impossible.
Bio:
Tadas currently leads the Human Synthetics team in Microsoft Cambridge. His primary research interests lie in the automatic understanding of human behavior, computer vision, and synthetic data generation. In particular, he is interested in the application of such technologies to virtual presence. He received his PhD and Bachelor’s degrees in Computer Science from Cambridge University and completed his post-doc at CMU.
Homepage: N/A
Sponsored in part by: Facebook Reality Labs Pittsburgh