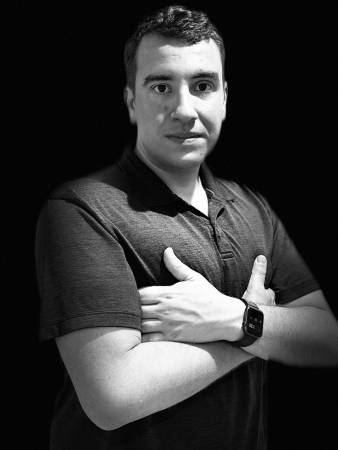
Miguel Angel Bautista
Staff Research Scientist
Apple Machine Learning Research
Monday, April 24
3:30 pm to 4:30 pm
Newell-Simon Hall 3305
3:30 pm to 4:30 pm
Newell-Simon Hall 3305
Generative modeling: from 3D scenes to fields and manifold
Abstract: In this keynote talk, we delve into some of our progress on generative models that are able to capture the distribution of intricate and realistic 3D scenes and fields. We explore a formulation of generative modeling that optimizes latent representations for disentangling radiance fields and camera poses, enabling both unconditional and conditional generation of 3D scenes. This approach achieves state-of-the-art performance across multiple datasets and allows for conditional generation based on sparse image observations or textual descriptions of the scene.
Furthermore, we will also discuss a recent diffusion model designed to learn distributions over fields. This model extends the formulation of vanilla diffusion probabilistic models to explicitly handle fields enabling an end-to-end learning algorithm that eliminates the requirement of representing fields with latent vectors. We showcase the model’s ability to effectively handle various modalities, including 2D images, 3D geometry, as well as fields defined on manifolds all while using the same network architecture.
Bio: Miguel Angel Bautista is a Research Scientist at Apple Machine Learning Research (MLR), where he carries out research in machine learning and computer vision. His current focus is on developing generative models that make as little assumptions about data as possible. Before joining Apple, he held a Postdoctoral position at Björn Ommer’s lab, where he led several research projects around self-supervision in deep learning, as well as the first forays into generative modeling (pre Stable Diffusion). Miguel got a Ph.D in Machine Learning in 2015 from University of Barcelona advised by Sergio Escalera and Oriol Pujol, working on learning Error-Correcting representations from data. Miguel spent a good part of his Ph.D at Carnegie Mellon University working with Fernando de la Torre on matrix factorization methods.
Sponsored in part by: Meta Reality Labs Pittsburgh