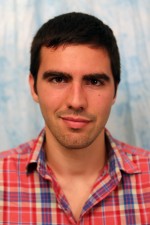
Carnegie Mellon University
12:30 pm to 2:00 pm
NSH 1109
Title: OpenPose: Whole-Body Pose Estimation
Abstract: We present the first single-network approach for 2D whole-body (body, face, hand, and foot) pose estimation, capable of detecting an arbitrary number of people from in-the-wild images. Our method maintains constant real-time performance regardless of the number of people in the image. This network is trained in a single stage using multi-task learning and an improved architecture, which account for the inherent scale difference between body/foot and face/hand keypoints. Our approach considerably improves upon the only known work in whole-body pose estimation (our previous work, OpenPose) in both speed and global accuracy. Unlike the original OpenPose, our new method does not need to run an additional network for each hand and face candidate, making it substantially faster for multi-person scenarios. This work directly results in a reduction of computational complexity for applications that require 2D whole-body information (e.g., re-targeting). In addition, it yields higher accuracy, especially for occluded, blurry, and low resolution faces and hands. Our code, trained models, and validation benchmarks will be publicly released as a baseline for future work in the area.
Committee:
Yaser Sheikh (advisor)
Kris Kitani
Aayush Bansal