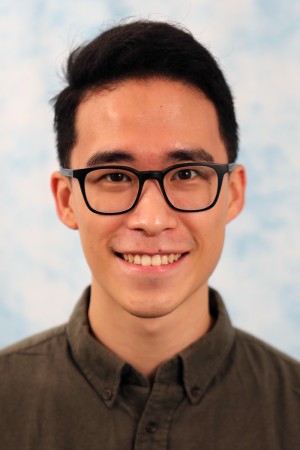
Abstract:
A large body of research exists on grasping for objects with ideal properties like Lambertian reflectance and rigidity. On the other hand, real-world environments contain many objects for which such properties do not hold, such as transparent, specular, and deformable objects. For such objects, new approaches are required to achieve the same level of grasping performance. First, we present a novel method for grasping transparent and specular objects. Our approach leverages the fact that transparent and reflective objects are easier to detect in the RGB modality than in depth. Based on this insight, we use cross-modal distillation to learn how to grasp transparent and specular objects, without requiring any real-world grasps or simulation of transparent or specular objects for training.
Second, we address the problem of grasping specific cloth regions for downstream manipulation. In many cloth-related tasks such as laundry folding and bed making, it is crucial to manipulate specific regions like cloth edges and corners, as opposed to wrinkles. We train a segmentation network to estimate the location of cloth edge and corner regions. Then, we propose an algorithm to select a grasp pose from the segmented regions, taking grasp direction and directional uncertainty of the segmented regions into account. In both projects, we show how we leverage our insights about the object properties to achieve state-of-the-art grasping performance.
Committee:
Prof. David Held (Chair)
Prof. Oliver Kroemer
Prof. Chris Atkeson
Mohit Sharma