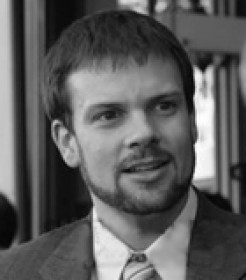
3:00 pm to 12:00 am
Event Location: NSH 3305
Bio: C. Lawrence Zitnick received the PhD degree in robotics from Carnegie Mellon University in 2003. His thesis focused on efficient inference algorithms for large-problem domains. Previously, his work centered on stereo vision, including the development of a commercial portable 3D camera. Currently, he is a researcher at the Interactive Visual Media group at Microsoft Research. His latest research includes object recognition and computational photography. He holds over 15 patents, and developed PhotoDNA to combat illegal imagery on the web.
Abstract: Humans and machines see the world differently, each having their own strengths and weaknesses. In this talk, I describe two projects exploring how they may help each other.
Visual object recognition by machines is notoriously difficult. To help in the learning process, humans are typically used to gather large hand-labeled training datasets from which the machines may learn. However, humans may also be used to “debug” the machine’s recognition pipeline to learn what aspects are lacking. Specifically, we explore the various stages of part-based person detectors. We perform human studies in which subjects perform the same sub-tasks as their machine counterparts, and accuracies are compared.
The typical human has significant difficultly in drawing everyday objects containing complex structures, such as faces or bikes. When learning to draw, humans must learn to see the word differently. That is, they must not only recognize what they are seeing, but they must perceive the spacing and structural layout of an object. We demonstrate an application in which machines can recognize what a human is drawing and provide visual guidance to the drawer in the form of shadows. The shadows, which may be either used or ignored by the drawer, help the drawer achieve more realistic overall shapes and spacing, while maintaining their own unique drawing style.