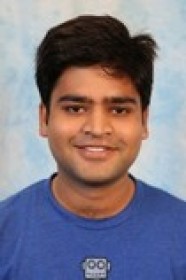
12:00 pm to 1:00 pm
Event Location: NSH 1507
Bio: Shobhit Srivastava is an M.S. student in the Robotics Institute at Carnegie Mellon University, advised by Prof. Nathan Michael. The primary focus of his research is to enable high-fidelity and efficient multimodal environment modeling on mobile autonomous systems to enable efficient inference with respect to the environment. He previously received his Bachelor’s degree in Computer Science and Engineering from National Institute of Technology, Allahabad, India and has worked for a couple of years at Qualcomm Research before coming to CMU for his Masters.
Abstract: Operation of mobile autonomous systems in real-world environments and their participation in the accomplishment of meaningful tasks requires a high-fidelity perceptual representation that enables efficient inference. It is challenging to reason efficiently in the space of sensor observations primarily due to the dependence of nature of measurements on the type of sensor, and in some cases, the prohibitive size of sensor data. A perceptual representation that abstracts out sensor nuances is thus required to enable effective and efficient reasoning in previously unknown environments.
In this talk, a probabilistic environment representation based on Hierarchical Gaussian Mixture Models that allows efficient high-fidelity modeling and inference towards enabling informed planning (active perception) on a computationally constrained mobile autonomous system, will be presented. An information-theoretic methodology to estimate the required model complexity and real-time viability on a computationally-constrained SoC via a GPU-based implementation will be discussed. An extension of the model to obtain a probabilistic representation of occupancy with an associated measure of uncertainty, via a regression framework, will also be presented.